Generative AI: Unlocking New Possibilities in Financial Services
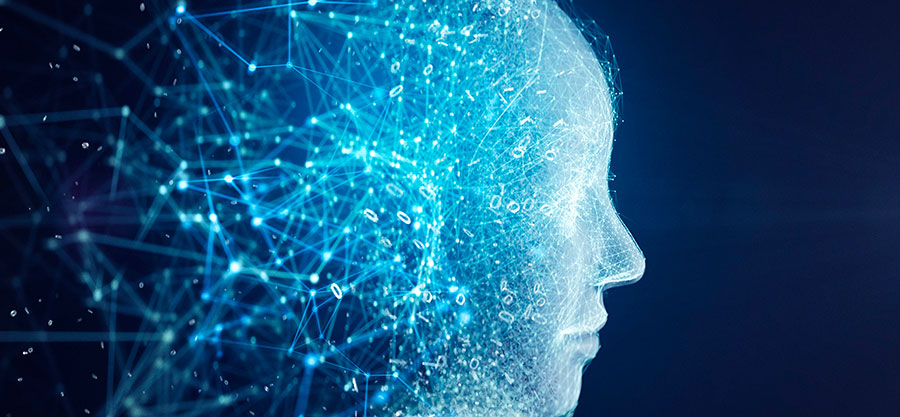
Generative AI—artificial intelligence that can create novel content such as text, images, code, and video—is all over the news right now. It seems like every day a new product or update takes the internet by storm, from the highly sophisticated chatbot ChatGPT to developer assistant Tabnine, which now generates 30-40% of its users’ code for them.
Generative AI is already impacting several inherently creative industries, with Jasper AI’s copywriting tool reaching around 100,000 users and generating $45MM in revenue in 2022. More broadly, many leading venture investors believe that it could spark the next “Cambrian explosion” of innovation across the tech ecosystem. But what can generative AI—and the fast-growing landscape of generative AI-based tools and startups—offer to financial services firms?
At Citi Ventures, we have long been believers and investors in AI-first companies such as Concentric, Deepgram, Eightfold, and Persado. As such, we have been asking those very questions ourselves for some time. Here are some of our findings.
The Evolution of Generative AI
Despite its recent emergence in the public sphere, the concept of generative AI is not new. Scientists have used its building blocks, including recurrent neural networks (RNNs), to generate outputs like text and images for years. However, for a long time these models produced low-quality outputs, were expensive to train, offered few commercial applications, and could only be accessed by a small fraction of developers.
That began to change in 2017, when Google Brain released a new type of neural architecture called a transformer. Unlike other AI models, transformer models are “data hungry” and can be adapted to downstream tasks—meaning that they can be trained on the entirety of Wikipedia then used for a variety of applications. For this reason, Stanford researchers dubbed transformers and other similar models foundation models.
In 2018, Google’s Bidirectional Encoder Representations from Transformers (BERT) model and OpenAI’s Generative Pre-trained Transformer (GPT) model catalyzed an explosion of transformer-based models that can create new content. As those models have matured and the number of model parameters has skyrocketed (e.g., GPT-2 had 1.5B; GPT-3 has 175B), they have become more accurate and have improved their generative abilities significantly. Currently, generative AI tools can produce work that resembles that of a middle schooler; with further maturation and training, the sky is the limit.
But perhaps the biggest leap in the growth of generative AI occurred in 2022, when developers worldwide gained access to foundation models that had previously only been available in research settings or limited private betas and were generally quite expensive. Thanks to companies like Hugging Face (which maintains an open-source transformers library), Stability AI (creator of publicly available open-source models such as Stable Diffusion), and OpenAI, developers can now utilize a wide range of powerful AI models to build myriad applications.
And they have done just that: as of January 2023, there are over 450 generative AI companies building solutions for numerous use cases spanning industries—including several of particular interest to financial services firms.
Generative AI Use Cases for Financial Services
Generative AI offers innumerable consumer and enterprise use cases. In fact, Sequoia Capital recently suggested that in “every industry that requires humans to create original work…generative AI can make these workers at least 10% more efficient and/or creative. …Therefore, generative AI has the potential to generate trillions of dollars of economic value.”
Generative AI offers innumerable consumer and enterprise use cases. In fact, Sequoia Capital recently suggested that in “every industry that reqWhile text-based applications are the most salient for enterprises as of now, video and image generation have gained widespread consumer interest as well. However, by and large these applications still create content that is recognizably AI-generated and is often inaccurate. Thus, generative AI is currently best suited for human-machine “co-creation,” such as AI-assisted copywriting.
Generative AI offers innumerable consumer and enterprise use cases. In fact, Sequoia Capital recently suggested that in “every industry that reqAt Citi Ventures, we are especially interested in generative AI startups that are tackling enterprise workflows applicable to financial services. The use cases we are most excited about as of now include:
- Search, Summarization, and Insights – E.G., enterprise search of private company documents; insight generation for equity research; and creating quarterly performance reports for private banking clients
- Sales and Marketing – E.G., personalized copy for marketing branded credit cards; personalized messaging; customer segmentation; marketing and sales analytics; and automated marketing campaigns
- Customer Engagement – E.G., chat bots; voice assistants; call center automation; real-time translation; customer analytics; sentiment and intent analysis; and agent support
- Legal Tech – E.G., generating and managing financial contracts; risk assessment of customer documents; and legal research
- Software Development – E.G., code generation; documentation; debugging; code refactoring; and code repository search
Lastly, we believe that there is further opportunity for growth among “model tooling” companies, which help other firms train, monitor, and/or deploy their own foundation models or adapt existing models for their specific use cases.
Here is an overview of areas and use cases we are excited about, including select companies within each space.
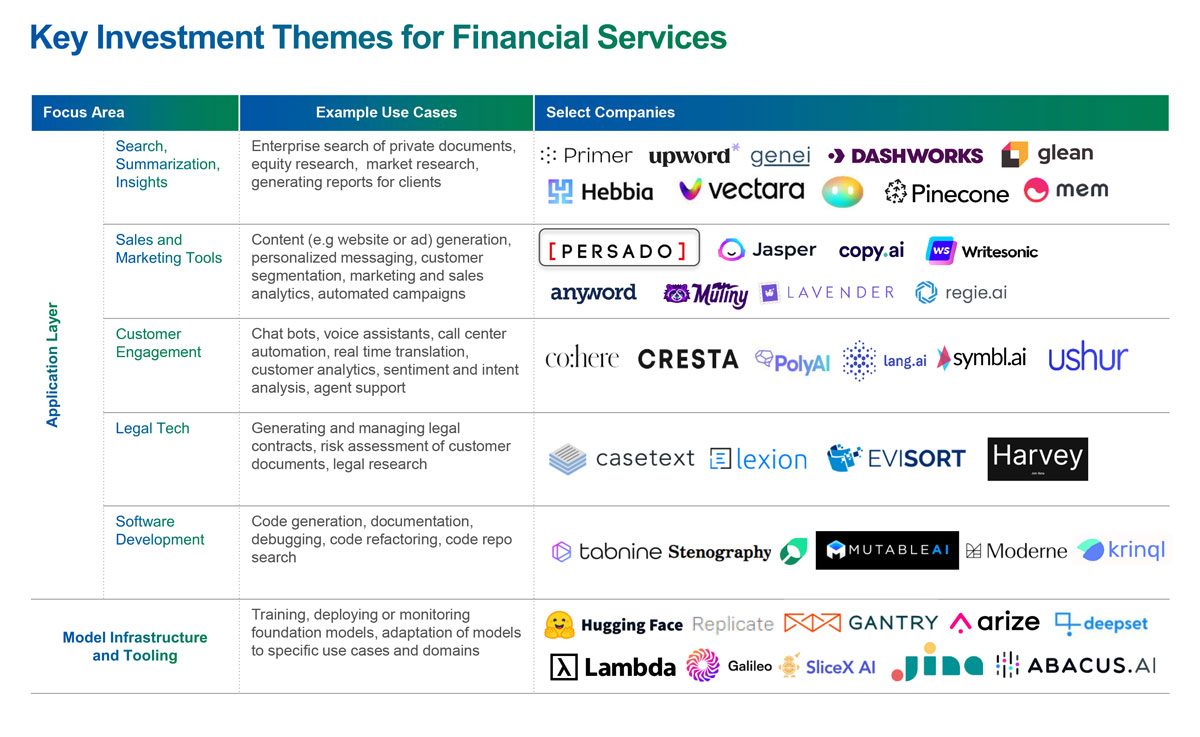
Generative AI Startup Landscape and Challenges to Growth
Given all this potential, it is no wonder that the generative AI ecosystem bucked 2022’s broader downward VC funding trend, with over $1.37B invested across 78 deals in 2022 and the market expected to grow significantly over the next few years. Even within the financial services-specific use cases we are exploring, there are already dozens of generative AI companies jockeying for market position—including Citi Ventures portfolio company Persado.
As the hype cycle reaches its peak, however, we are keeping our eyes on potential obstacles to growth as well. These include:
- Making the leap from consumer-grade to enterprise-grade tech – As noted, many generative AI use cases are not yet ready for enterprise adoption. We expect more enterprise-grade tools to arrive within approximately 2-4 years.
- Defensibility and moat for app-layer companies – Since many application-layer generative AI companies are built on top of the same foundation models (e.g., GPT-3), they will need an additional competitive moat in order to get ahead. Network effects, unique distribution, and platform capabilities are some ways these companies might build long-term defensibility.
- Platform dependency – The fact that many app-layer companies rest on the same model layer also raises significant concerns about platform dependency—if a foundation model company such as OpenAI changes its fee structure or (worse) stops supporting certain use cases, the companies built on top of it could struggle to survive. However, we believe that models will become more commoditized/exchangeable in the upcoming years, which should minimize this dependency.
- Copyright and IP risk – Generative AI brings with it copyright and other intellectual property concerns that have yet to be fleshed out and could pose a major risk to companies in the space. For example, we still do not know the copyright implications around input data used to train foundation models, nor whether one can copyright the output of these models. A class-action lawsuit has been filed against GitHub, Microsoft, and OpenAI regarding GitHub Copilot, and Getty Images recently sued Stability AI for unlawfully scraping content from its site. As with any new technology, we will keep close tabs on how these lawsuits evolve and how the legal system adapts over time.
Despite these challenges, we expect the generative AI space to continue moving forward rapidly: with so many companies emerging and so much capital flowing in, both the model and application layers are improving at a speed we haven’t seen in many other industries. As the landscape continues to shift, we believe that the team itself (their unique know-how and ability to move fast) will prove to be the most important part of the new companies coming into the space.
Generating Game-Changing Innovation in Financial Services
From deducing regulatory risk from financial documents to corresponding with banking customers, we at Citi Ventures believe that generative AI has the potential to transform the financial services industry in never-before-seen ways. We look forward to tracking the growth of this dynamic field, investing in the companies that will come to define it, and perhaps one day using generative AI tools to help write these articles.
For more information, email Cagla Kaymaz at cagla.kaymaz@citi.com, Matt Carbonara at matt.carbonara@citi.com, Vibhor Rastogi at vibhor.rastogi@citi.com, or Avi Arnon at avi.arnon@citi.com.