Automated Process Discovery: Smarter Automation through AI
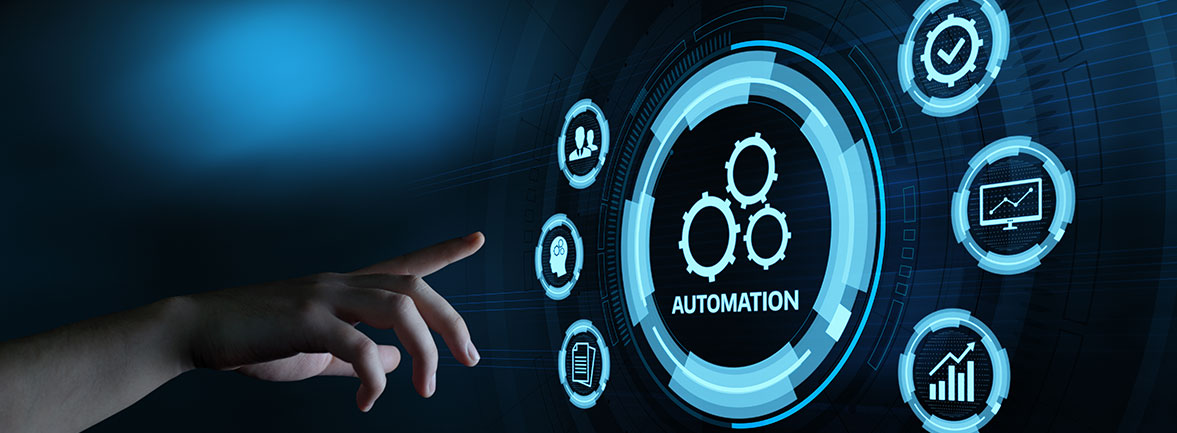
Robotic Process Automation (RPA), the use of software tools to replace human workers on mundane and repetitive data-based tasks, is becoming a key element of digital transformation efforts in the financial industry and elsewhere.
Promising to reduce efforts and costs, increase productivity and profits, and free up employees for higher-value work, RPA was the fastest-growing software segment in 2018 and is expected to grow from a $1.6 billion to a $46 billion global market by 2030. Moreover, the coronavirus pandemic is likely to increase the rate of RPA adoption as organizations accelerate digital transformation efforts.
However, implementing RPA can be challenging, as initiatives often are unable to scale from project to enterprise-level or to deliver a promised ROI. Ernst & Young, which has delivered RPA projects across 20 countries, reports that it has seen 30 to 50 percent of initial projects fail. These failures occur less because of faulty technology than from targeting the wrong processes for transformation. And that raises a crucial question: how can organizations determine which of their processes are right for RPA?
For the answer, companies are turning to Automated Process Discovery (APD), which uses AI and machine learning (ML) to identify, analyze, and map the underlying structure and processes of day-to-day business operations. APD collects and sorts data on how workers use various systems and applications to perform their tasks. This helps organizations more quickly, comprehensively, and cost-effectively evaluate the automation and re-engineering potential of their processes.
These assessments enable companies to implement new and improved processes that enhance the customer journey, make product and service delivery more agile, and allow employees to be more efficient.
A Longtime Goal
For decades, organizations have sought to better understand their own processes by having analysts and consultants review company documents, watch employees perform tasks, and interview those employees about their workday activities and routines. This approach has drawbacks. It can be time-consuming, human capital-intensive, distracting to daily operations, and prone to biases and guesswork. It also provides an individualized and incomplete view of complex and interconnected processes that can span the breadth of an enterprise.
In the late 2000s, the new field of process mining enabled faster and a more thorough view of organizational workflows and procedures by using software to collect and analyze data from the system logs of back-end Customer Relationship Management (CRM) and Enterprise Resource Planning (ERP) systems. But because process mining does not read data from user-level activities that occur outside of a firm's major applications—like using a word processing program or a virtual environment—its utility is constrained.
APD replaces traditional analysis and complements process mining by working in the background to record user activity across multiple applications and systems, even taking screenshots to gain insight into how work is done from the user's perspective. Once that data is collected, AI and ML are used to detect commonly occurring patterns and infer broader process structure.
Taking Action
As a relatively new and quickly evolving way to improve on traditional methods of organizational self-evaluation, APD has great potential to help firms make faster and better decisions about which internal processes to automate, re-engineer, or otherwise optimize. This can increase productivity and profitability at levels of scale that previously were impractical.
Healthcare companies, for example, could improve outcomes for patients by making treatment pathways more efficient, while manufacturers could improve procurement and production by identifying and resolving the causes of delays. An information and communication technology firm reported that APD helped one of its clients, a multinational energy company, identify the root causes of costly delays in the maintenance of its oil sands plants.
For large financial institutions, APD promises to improve internal process efficiency and customer experiences in many ways—including faster loan processing and credit checks—while helping the company determine when and how to use RPA. For organizations engaged in digital transformation, RPA promises significant ROI—provided the right processes are optimized and automated.
APD can help firms identify the most promising targets and better understand their internal workings in order to drive continuous improvement.